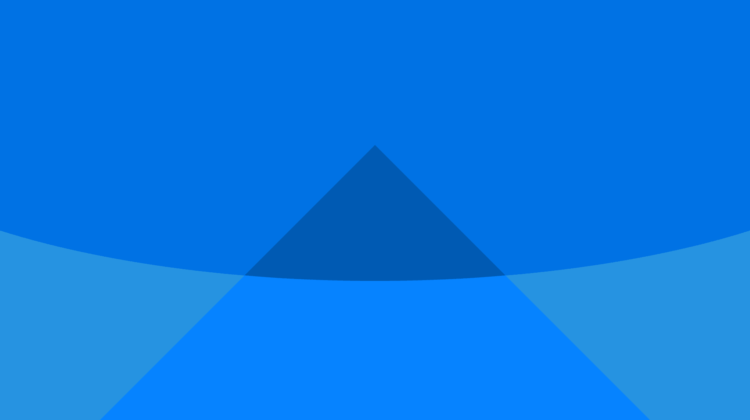
The Metamorphosis of Economic Prediction: From Conventional Approaches to Machine Learning
The field of economic prediction has perpetually stood as a pivotal facet of contemporary economics, offering sagacious guidance for policy formulation, corporate planning, and investment strategies. The annals of time have witnessed a profound transformation in the methodologies and instruments employed for economic prognosis, mirroring the evolution in data accumulation, computational potency, and analytical methodologies. In this investigative exposition, we shall embark on an odyssey through the captivating narrative of economic prediction, tracing its roots in tradition to the groundbreaking epoch of machine learning.
Conventional Methods of Economic Prediction:
The history of economic forecasting is an extended narrative that harks back to the earliest human civilizations, relying on rudimentary models and anecdotal testimony to foresee agricultural yields, trading trends, and other economic phenomena. Nevertheless, it was not until the 20th century that more structured and quantitative methodologies began to take shape.
Temporal Sequence Scrutiny:
One of the foundational methods in economic prediction resides in temporal sequence scrutiny. This methodology necessitates a meticulous examination of historical data points, unravelling patterns, trends, and cyclical oscillations. Analysts employ techniques such as moving averages, autoregressive integrated moving average (ARIMA) models, and exponential smoothing to extrapolate future developments grounded in historical data.
Economic Barometers:
Economists and policymakers have perennially leaned on pivotal economic barometers to prophesy economic trajectories. These indicators encompass metrics like GDP growth rates, unemployment figures, inflation rates, and consumer sentiment. Alterations in these indicators frequently unveil invaluable insights into the economic well-being of a nation.
Econometric Frameworks:
Econometric frameworks, such as the Phillips Curve and the IS-LM model, gained ascendancy during the mid-20th century. These frameworks aspired to encapsulate the intricate interplay among economic variables, offering a theoretical scaffold for prediction grounded in mathematical equations.
Inherent Limitations of Traditional Approaches:
Traditional techniques in economic prediction, notwithstanding their historical significance, are not devoid of constraints. They frequently grapple with unforeseen and abrupt disruptions, as well as the intricate entanglement of variables. Additionally, they exhibit an acute dependence on historical data, rendering them less efficacious in mercurial and uncertain circumstances.
The Ascendancy of Machine Learning in Economic Forecasting:
The emergence of machine learning heralds a novel epoch in economic prediction, promising heightened precision and adaptability. Machine learning methodologies, underpinned by vast datasets and sophisticated algorithms, hold the potential to metamorphose the landscape of economic forecasting.
The Terrain of Big Data and Data Analytics:
Machine learning harnesses the prowess of big data analytics to expedite the processing and interpretation of colossal datasets. This capability empowers economists and analysts to incorporate an expansive gamut of variables and real-time data streams, including sentiment analysis from social media and online transactional data, into their predictive models.
Prognostic Analytics:
Machine learning algorithms, spanning neural networks, decision trees, and support vector machines, possess the ability to unearth intricate patterns and correlations within data, often concealed from conventional methodologies. This augmentation culminates in heightened precision in economic forecasts and a more holistic comprehension of economic dynamics.
Sentiment Scrutiny:
Machine learning algorithms can dissect sentiment data gleaned from news articles, social media platforms, and sundry sources to assess public perception and sentiment concerning the economy. This information can be invaluable in forecasting market responses and consumer comportment.
Challenges and Deliberations:
While machine learning bears great potential for economic prediction, it is not devoid of challenges. The opaque nature of certain machine learning models can complicate the interpretation of their forecasts, precipitating concerns regarding transparency and accountability. Furthermore, machine learning models necessitate copious volumes of high-quality data and substantial computational resources, which may not be universally accessible to all economic analysts.
The Prospects of Economic Prediction:
The evolution of economic prediction is ongoing, with traditional methods and machine learning paradigms coalescing and synergizing. In the foreseeable future, we can anticipate a hybrid approach where economists and analysts amalgamate the strengths of both traditional and machine learning methodologies to yield forecasts that are more precise and robust.
Epilogue:
The voyage of economic prediction has traversed an extensive path from its nascent days, replete with rudimentary practices and intuition-guided prognostications. The assimilation of machine learning into the realm of economic prediction signifies a momentous milestone, offering the potential for forecasts that are more precise, data-driven, and adaptable. As technology continues its relentless march forward, the panorama of economic prediction will continue its metamorphosis, sculpting the contours of informed decision-making in an ever-evolving economic milieu.
Author: Harvey Graham
Forecast analysis consultant in Great Britain. Collaborates with The Deeping in the economic forecasting area