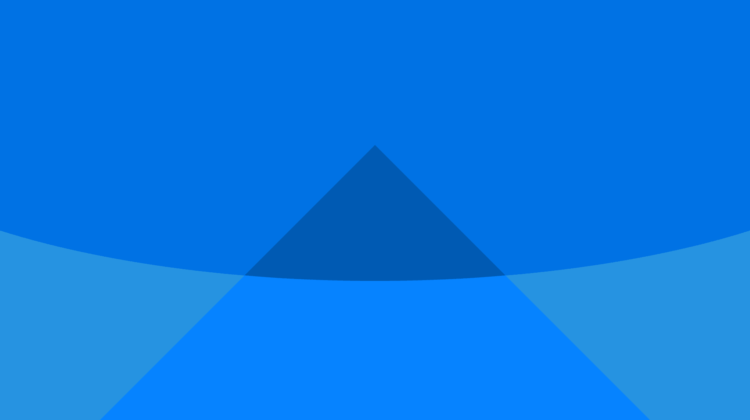
The Power of Prediction: Economic Forecasting in Business
In the realm of business, the ability to predict future economic conditions is akin to possessing a crystal ball. Economic forecasting, the practice of making predictions about future economic activity based on current and historical data, has become an indispensable tool for businesses seeking to navigate the complexities of the global economy.
This practice, grounded in statistical models and econometric techniques, allows businesses to make informed decisions, mitigate risks, and capitalise on opportunities. However, the power of prediction comes with its own set of challenges and ethical considerations, making it a sophisticated and sometimes contentious aspect of business strategy.
Economic forecasting encompasses a wide array of methodologies, each with its own strengths and limitations.
At its core, forecasting involves the analysis of various economic indicators such as GDP growth rates, inflation, unemployment, consumer spending, and business investment. These indicators provide valuable insights into the overall health of an economy and can be used to predict future trends. For instance, a consistent increase in consumer spending might signal robust economic growth, whereas rising unemployment rates could indicate an impending recession.
Forecasters employ a range of models to interpret these indicators, from simple trend analysis and moving averages to more complex methods such as autoregressive integrated moving average (ARIMA) models and vector autoregression (VAR) models. These models are often enhanced by incorporating qualitative data, such as expert opinions and market sentiment, to provide a more comprehensive view of future economic conditions.
One of the most widely used approaches in economic forecasting is the econometric model, which uses mathematical equations to describe the relationships between different economic variables.
These models can range from single-equation models, which focus on one specific aspect of the economy, to large-scale macroeconomic models that encompass multiple sectors and interactions. The latter, often used by central banks and government agencies, can simulate the effects of various policy changes and external shocks on the economy. For businesses, these models are invaluable for strategic planning and risk management. By understanding potential future scenarios, companies can make better decisions regarding investment, production, staffing, and marketing. For example, a manufacturing firm might use economic forecasts to anticipate changes in demand for its products and adjust its production schedules accordingly. Similarly, a multinational corporation might rely on forecasts to assess the potential impact of currency fluctuations on its international operations and develop hedging strategies to protect against adverse movements.
Despite the sophistication of modern forecasting techniques, the inherent uncertainty of the future means that predictions are never foolproof. The accuracy of economic forecasts depends on the quality and completeness of the data, the appropriateness of the chosen model, and the forecaster’s ability to account for unforeseen events.
The global financial crisis of 2008 is a stark reminder of the limitations of economic forecasting. Few economists predicted the scale and severity of the crisis, and many models failed to capture the complex interplay of factors that led to the economic meltdown. This has led to increased scrutiny of forecasting practices and a push for more robust and adaptive models.
One approach that has gained traction in recent years is the use of machine learning and artificial intelligence (AI) in economic forecasting. These technologies can process vast amounts of data at unprecedented speeds, identify patterns and correlations that traditional models might miss, and continuously improve their predictions through iterative learning. For instance, AI algorithms can analyse social media trends, news articles, and other unstructured data sources to gauge market sentiment and predict economic turning points.
While AI holds great promise, it also raises ethical and practical concerns. The reliance on complex algorithms can create a “black box” effect, where the decision-making process becomes opaque and difficult to scrutinise. This lack of transparency can undermine trust in the forecasts and lead to decisions that are not fully understood by human managers.
Additionally, the use of personal data in AI-driven forecasts raises privacy issues and necessitates stringent data protection measures.
The interplay between economic forecasting and public policy further complicates the landscape. Governments and central banks rely on forecasts to design and implement policies aimed at stabilising the economy, promoting growth, and controlling inflation. The accuracy of these forecasts is crucial, as policy missteps can have far-reaching consequences for businesses and households. For instance, an overly optimistic forecast might lead to premature tightening of monetary policy, stifling economic recovery. Conversely, an unduly pessimistic outlook could result in excessive fiscal stimulus, leading to inflationary pressures and unsustainable debt levels.
The challenge for policymakers is to balance short-term economic objectives with long-term stability, a task that requires not only accurate forecasts but also a deep understanding of the underlying economic dynamics.
The role of communication in economic forecasting cannot be overstated. Forecasts must be clearly communicated to stakeholders, including business leaders, investors, policymakers, and the general public. Effective communication involves not only presenting the predictions but also explaining the assumptions, methodologies, and potential uncertainties. Transparency in communication helps build trust and allows stakeholders to make informed decisions based on the forecasts.
However, the communication of economic forecasts is fraught with challenges. Forecasters must strike a delicate balance between providing actionable insights and avoiding undue alarm or complacency. Overly optimistic forecasts can lead to overinvestment and asset bubbles, while excessively pessimistic projections can dampen consumer and business confidence, exacerbating economic downturns. Moreover, the media’s portrayal of economic forecasts can influence public perception and behaviour, amplifying the effects of the forecasts themselves.
In conclusion, economic forecasting is a powerful tool that plays a vital role in business strategy and public policy. The ability to anticipate future economic conditions enables businesses to navigate uncertainty, seize opportunities, and mitigate risks. However, the practice of forecasting is inherently complex and fraught with challenges. Forecasters must grapple with the limitations of their models, the unpredictability of economic events, and the ethical implications of their work. As technologies such as AI and machine learning continue to evolve, the potential for more accurate and insightful forecasts grows. Yet, these advancements also bring new challenges, including the need for transparency, accountability, and data privacy.
Ultimately, the power of prediction lies not only in the accuracy of the forecasts but also in the wisdom and integrity with which they are used. As businesses and policymakers strive to harness the potential of economic forecasting, they must remain mindful of its limitations and ethical considerations, ensuring that their decisions are informed, responsible, and conducive to long-term prosperity and stability.
Author: Harvey Graham
Forecast analysis consultant in Great Britain. Collaborates with The Deeping in the economic forecasting area